
Photo by Tara Winstead on <a href="https://www.pexels.com/photo/an-artificial-intelligence-illustration-on-the-wall-8849295/" rel="nofollow">Pexels.com</a>
Artificial Intelligence (AI) has become a ubiquitous presence in our lives, from recommending products on e-commerce sites to powering the latest autonomous vehicles. However, as AI becomes more prevalent, there is a growing concern about its accountability and transparency. That’s where Explainable AI (XAI) comes into play. In this blog post, we’ll explore what Explainable AI is and its importance in making AI systems more transparent and trustworthy.
What is Explainable AI (XAI)?
Explainable AI (XAI) is an approach to AI development that seeks to create models and systems whose outputs can be easily understood by humans. The goal of XAI is to provide a clear and concise explanation of how an AI system arrived at a particular decision. This is particularly important for complex AI systems that are based on machine learning algorithms, which can be difficult to interpret.
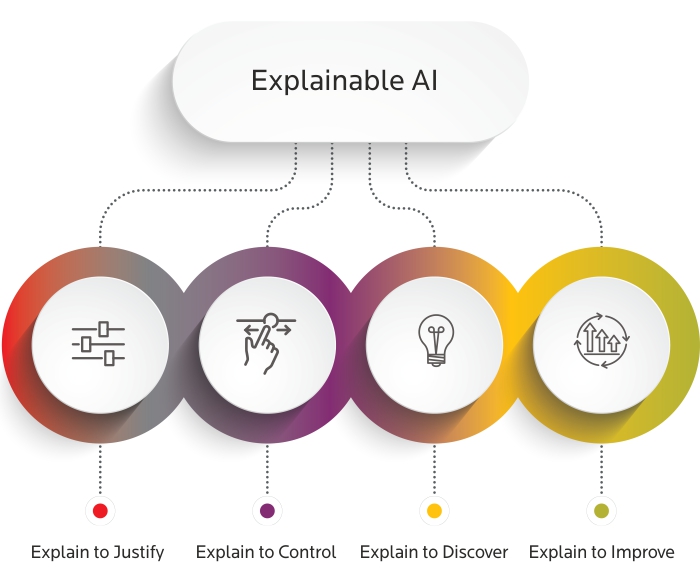
Why is XAI important?
There are several reasons why XAI is important. One of the primary concerns with AI systems is that they can make decisions that are biased or unfair. This is especially true for systems that are trained on large datasets, which may contain implicit biases. XAI can help address this issue by providing insight into how an AI system arrived at a particular decision, allowing for bias to be identified and corrected.
Another reason why XAI is important is that it can help build trust in AI systems. When humans can understand how an AI system arrived at a decision, they are more likely to trust the system. This is particularly important in industries such as healthcare and finance, where decisions made by AI systems can have significant consequences.
XAI can also help improve the performance of AI systems. By providing insight into how a system arrived at a particular decision, developers can identify areas where the system can be improved. This can lead to more accurate and efficient AI systems.
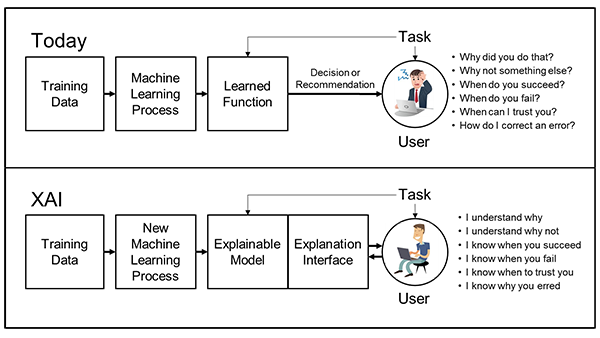
Examples of XAI in Action
One example of XAI in action is the Google Cloud AutoML Vision system. This system allows developers to build custom image recognition models without any prior machine learning knowledge. The system also provides explanations for how it arrived at a particular decision, making it easier for developers to understand and improve the system.
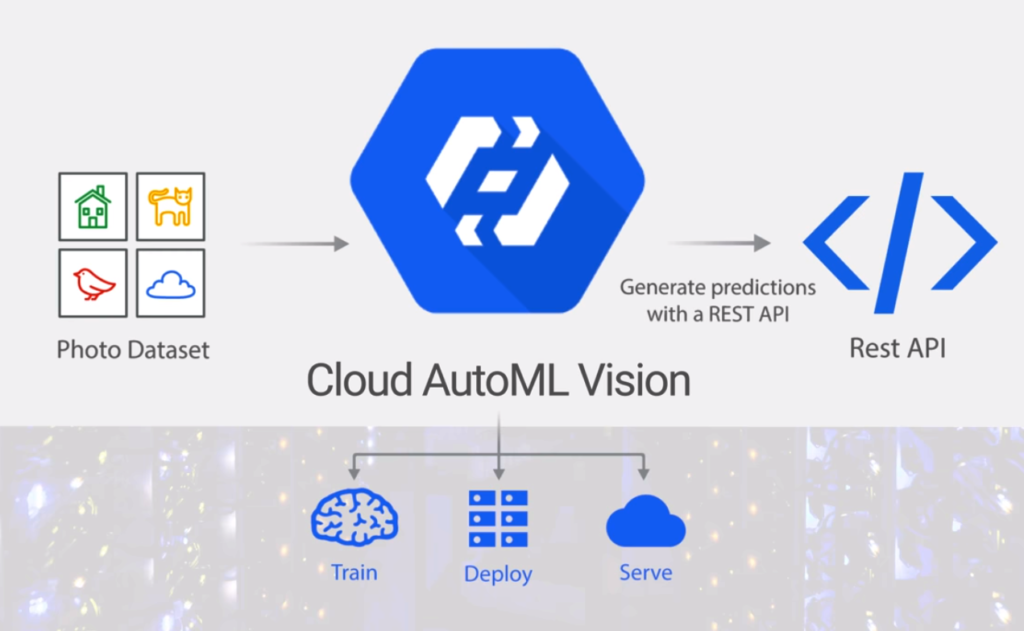
Another example is the Explainable AI Toolkit developed by IBM. This toolkit provides developers with tools for building explainable AI systems, including techniques for interpreting machine learning models and visualizing their outputs.
Conclusion
Explainable AI (XAI) is an important development in the world of AI. It can help address issues of bias and improve the transparency and trustworthiness of AI systems. As AI becomes more prevalent in our lives, XAI will play an increasingly important role in ensuring that these systems are accountable and transparent.