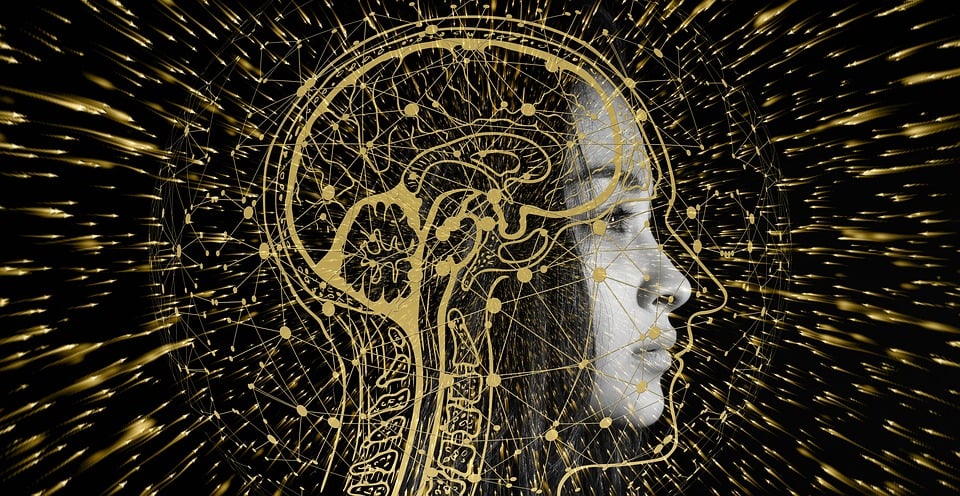
Explore the fascinating intersection of quantum computing and artificial intelligence, and how this powerful combination is shaping our world.
Introduction
Quantum computing and artificial intelligence (AI) have been making headlines in recent years, and for good reason. These two groundbreaking technologies are not only redefining the limits of computation and human-machine interaction but also promising to revolutionize industries across the globe. In this blog post, we’ll delve into the fascinating world of quantum computing and AI, explore their potential synergy, and uncover how this powerful combination is driving innovation in various sectors.
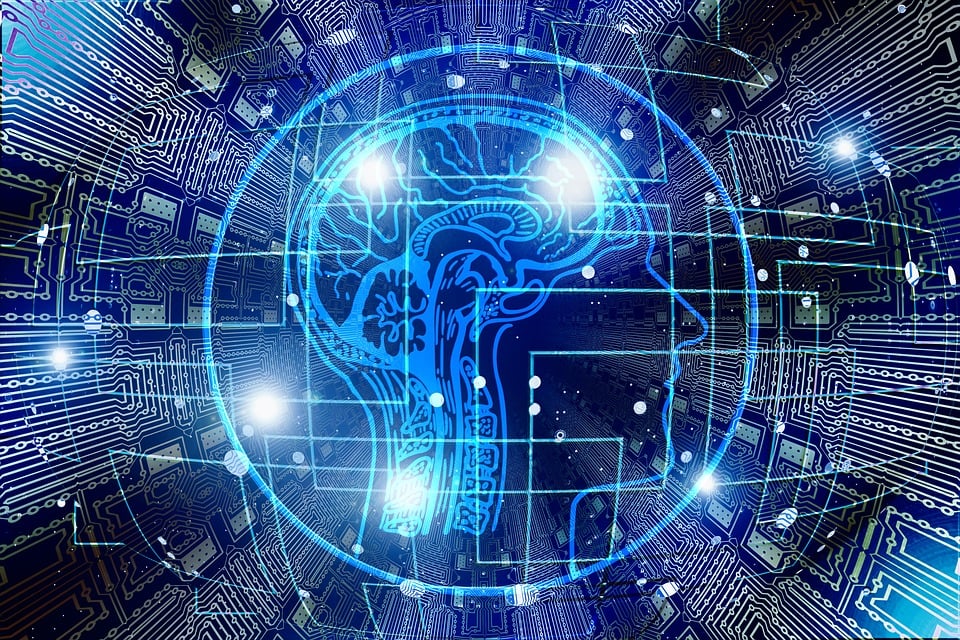
The Quantum Leap: A Brief Overview of Quantum Computing
Quantum computing harnesses the principles of quantum mechanics to perform complex calculations at unprecedented speeds. Unlike classical computers, which use bits as the smallest unit of data (either a 0 or a 1), quantum computers utilize quantum bits or qubits. Qubits can exist in multiple states simultaneously, thanks to a phenomenon called superposition. This unique property allows quantum computers to process vast amounts of information in parallel, making them exponentially faster than their classical counterparts for certain tasks1.
Artificial Intelligence: Decoding the Human-Machine Symbiosis
AI, on the other hand, is the development of computer systems that can perform tasks that typically require human intelligence. This includes problem-solving, learning, planning, and understanding natural language. AI systems leverage machine learning algorithms to analyze massive amounts of data, identify patterns, and make predictions or decisions based on that analysis2.
The Synergy: Quantum Computing and AI Unleashed
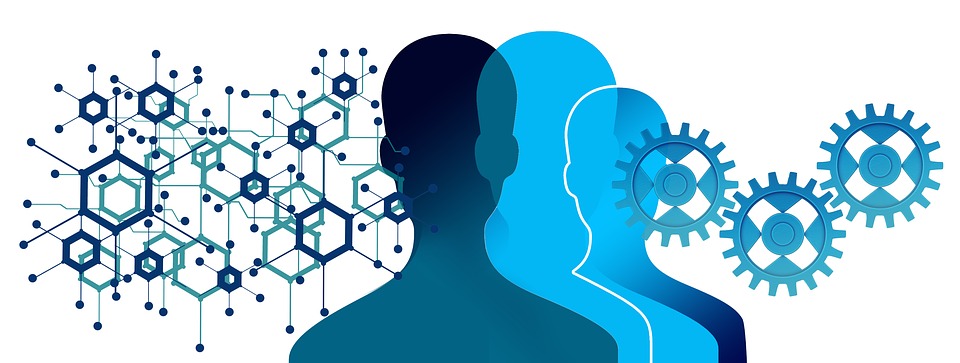
The convergence of quantum computing and AI could lead to significant breakthroughs in various fields. Quantum-enhanced machine learning algorithms have the potential to solve complex problems that are currently intractable for classical computers. By incorporating quantum principles into AI systems, researchers aim to:
- Accelerate training and optimization of AI models: Quantum computers can process large datasets more efficiently than classical computers, potentially reducing the time needed to train AI models and optimize their performance[^3^].
- Solve complex optimization problems: Many real-world problems, such as traffic management, drug discovery, and supply chain optimization, can be modeled as optimization problems. Quantum computing can help find optimal solutions to these problems faster than classical methods[^4^].
- Enhance AI security: Quantum computing can improve the security of AI systems by introducing quantum encryption techniques, making them less susceptible to cyberattacks[^5^].
Examples of Quantum Computing and AI in Action
Several projects are already underway that explore the intersection of quantum computing and AI. Some notable examples include:
- Google’s Quantum AI team is researching quantum algorithms for machine learning, optimization, and cryptography[^6^].
- IBM has developed the Qiskit software framework, which allows researchers to create and simulate quantum computing programs, including those related to AI[^7^].
- Volkswagen has partnered with D-Wave Systems to develop a traffic management system that utilizes quantum computing to optimize urban traffic flow[^8^].
The Future of Quantum Computing and AI
- Drug discovery and personalized medicine: Quantum-enhanced AI can potentially revolutionize drug discovery by accelerating the identification of new compounds and their interactions with biological targets. It may also enable personalized medicine by predicting an individual’s response to specific treatments based on their genetic information1.
- Climate modeling and environmental forecasting: The combination of quantum computing and AI can lead to more accurate climate models and predictions, which may help mitigate the effects of climate change and better inform environmental policy2.
- Financial markets and portfolio optimization: Quantum computing can help optimize financial portfolios, enhance risk assessment, and improve trading strategies by processing vast amounts of financial data and complex market variables3.
- Advanced robotics and autonomous systems: Quantum-enhanced AI algorithms could enable the development of more advanced and adaptive robotic systems, capable of performing complex tasks in dynamic environments with greater efficiency[^12^].
As we continue to explore the synergy between quantum computing and AI, we can expect to witness transformative advances that reshape our world and redefine what is possible.
Emerging Challenges and Ethical Considerations
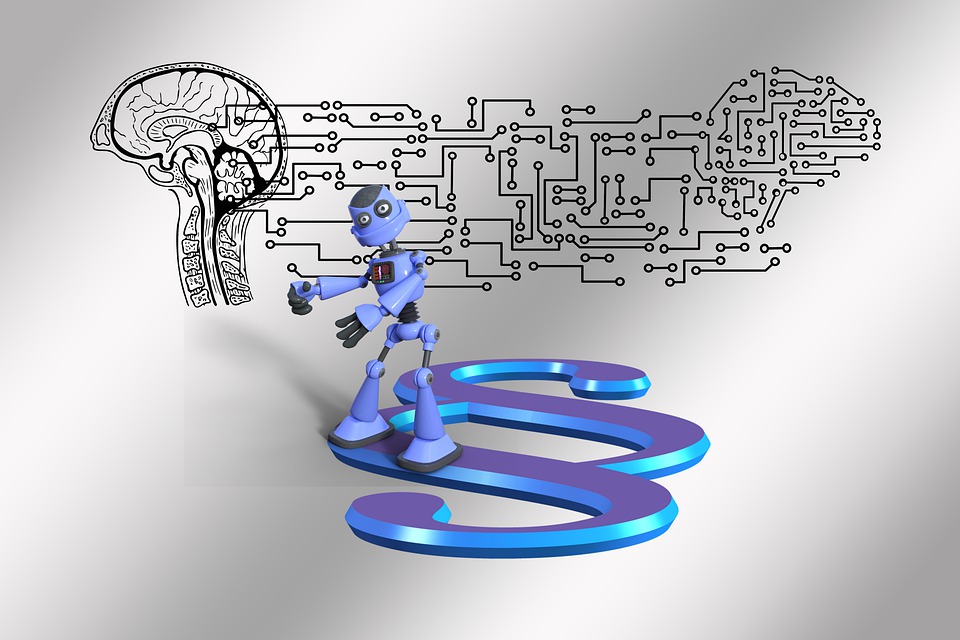
As the synergy between quantum computing and AI continues to unfold, it is essential to consider the potential challenges and ethical implications associated with their development and implementation. Some areas of concern include:
- Data privacy and security: The rapid advancements in quantum computing could potentially render current encryption methods obsolete, posing significant risks to data privacy and security. It is crucial to develop and adopt new quantum-resistant encryption techniques to safeguard sensitive information and maintain trust in digital systems[^5^].
- Quantum supremacy and its implications: Achieving quantum supremacy, where a quantum computer can outperform the most powerful classical computer in specific tasks, could disrupt various industries and create an uneven playing field. There is a need for international collaboration and regulatory frameworks to ensure the responsible development and deployment of quantum technologies[^13^].
- Bias and fairness in AI: As AI systems rely on large datasets for training, they may inadvertently perpetuate existing biases in the data. Quantum-enhanced AI models must be developed with fairness and transparency in mind to avoid exacerbating inequality and discrimination[^14^].
- Job displacement and workforce development: The integration of quantum computing and AI could lead to job displacement in certain sectors. Governments, educational institutions, and industries must collaborate to develop effective strategies for reskilling and upskilling the workforce to meet the demands of a quantum-AI-driven future[^15^].
As we continue to unlock the potential of quantum computing and AI, addressing these challenges and ethical considerations is vital to ensure that their benefits are realized and shared equitably across society.
Conclusion
As quantum computing and AI continue to advance, their synergy is expected to unlock new possibilities and drive innovation across various industries. From enhancing machine learning capabilities to solving complex optimization problems and improving AI security, the potential applications of this powerful combination are both exciting and vast. Stay tuned to our blog for more updates on the fascinating world of quantum computing and AI.
References:
[1]: Nielsen, M. A., & Chuang, I. L. (2010). Quantum Computation and Quantum Information: 10th Anniversary Edition. Cambridge University Press.
[2]: Russell, S. J., & Norvig, P. (2016). Artificial Intelligence: A Modern Approach (3rd ed.). Pearson Education.
[3]: Biamonte, J., Wittek, P., Pancotti, N., Rebentrost, P., Wiebe, N., & Lloyd, S. (2017). Nature, 549(7671), 195–202. Quantum Machine Learning. https://doi.org/10.1038/nature23474
[4]: Preskill, J. (2018). Quantum, 2, 79. Quantum Computing in the NISQ Era and Beyond. https://doi.org/10.22331/q-2018-08-06-79
[5]: Shor, P. W. (1997). SIAM Journal on Computing, 26(5), 1484-1509. Polynomial-Time Algorithms for Prime Factorization and Discrete Logarithms on a Quantum Computer. https://doi.org/10.1137/S0097539795293172
[6]: Google AI Quantum. (n.d.). Retrieved from https://research.google/teams/applied-science/quantum-ai/
[7]: IBM Quantum. (n.d.). Retrieved from https://www.ibm.com/quantum-computing/
[9]: Cao, Y., Romero, J., Olson, J. P., Degroote, M., Johnson, P. D., Kieferová, M., … & Aspuru-Guzik, A. (2018). Chemical Reviews, 119(19), 10856-10915. Quantum Chemistry in the Age of Quantum Computing. https://doi.org/10.1021/acs.chemrev.8b00803
[10]: Preskill, J., & Krishnan, S. (2021). AGU Advances, 2(4), e2021AV000416. Quantum Computing in the NISQ Era and Beyond: Prospects for Climate Science. https://doi.org/10.1029/2021AV000416
[11]: Orús, R., Mugel, S., & Lizuain, I. (2019). Reviews in Physics, 4, 100028. Quantum Computing for Finance: Overview and Prospects.
[12]: Hutter, M., & Legg, S. (2006). Robotics and Autonomous Systems, 54(9), 772-791. A Collection of Definitions of Intelligence. https://doi.org/10.1016/j.robot.2006.03.006
[13]: Montanaro, A. (2016). NPJ Quantum Information, 2, 15023. Quantum Algorithms: An Overview. https://doi.org/10.1038/npjqi.2015.23
[14]: Mehrabi, N., Morstatter, F., Saxena, N., Lerman, K., & Galstyan, A. (2019). arXiv:1902.03531. A Survey on Bias and Fairness in Machine Learning. Retrieved from https://arxiv.org/abs/1902.03531
[15]: Arntz, M., Gregory, T., & Zierahn, U. (2016). OECD Social, Employment and Migration Working Papers, No. 189. The Risk of Automation for Jobs in OECD Countries: A Comparative Analysis. http://dx.doi.org/10.1787/5jlz9h56dvq7-en